Beyond Single Tasks: The Compelling Case for Multi-Agent Systems
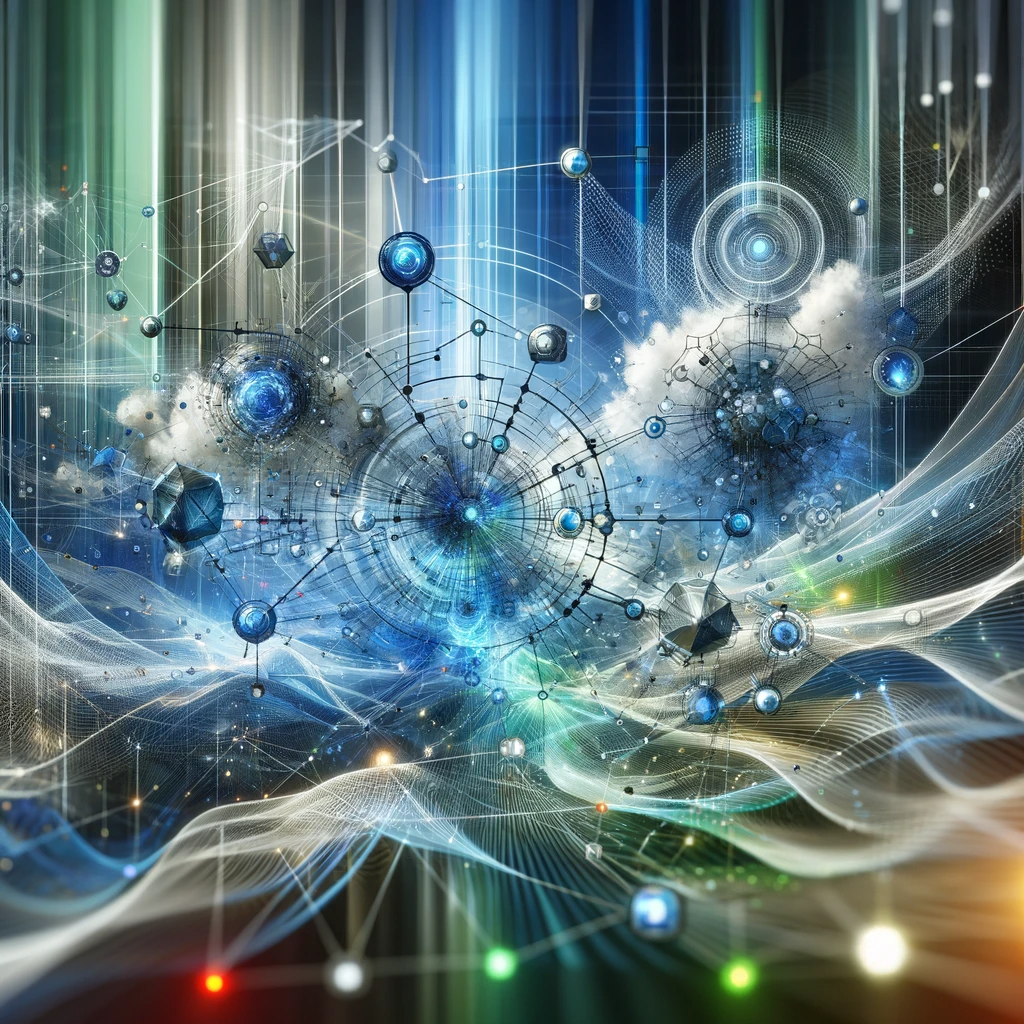
Introduction to Multi-Agent Systems and Current AI Trends
Overview of Multi-Agent Systems in the Context of Generative AI
The paradigm shift towards generative AI has significantly amplified the importance of multi-agent systems in the enterprise sector. Unlike traditional AI models that focus on single-task executions, multi-agent systems comprise numerous AI entities working in tandem, facilitating more complex and nuanced operations. This approach aligns perfectly with the evolving landscape of generative AI, where versatility and collaborative problem-solving are paramount. As businesses adopt Generative AI to enhance productivity and reshape their operational frameworks, multi-agent systems emerge as a vital cog in this transformative journey. Their ability to coordinate multiple generative AI models and tools positions them as a powerful solution for intricate business processes and decision-making scenarios.
Discussion of the 2023 AI Trends
Generative AI has taken a front seat in technological discussions, revolutionizing productivity for developers and knowledge workers. It's driving organizations to reassess their business processes and the role of human resources. Critical technologies like cloud AI services, computer vision, data-centric AI, and edge AI are pivotal in this landscape. These technologies not only fuel the advancements in Generative AI but also benefit from its progression.
In the enterprise sector, multi-agent systems are increasingly recognized for their strategic value. These systems allow for a more dynamic and flexible approach to AI implementation, accommodating the complex and ever-evolving business environments. They offer a structured way to deploy and manage various AI models and tools, making them indispensable for organizations aiming to leverage AI for comprehensive, multi-dimensional solutions.
Advantages of Multi-Agent Systems in Complex Environments
Multi-agent systems outshine single AI entities in complex environments by coordinating multiple generative AI models, plugins, and tools. These systems adeptly assume complex organizational roles, automating collaboration for solving intricate tasks. This results in superior business outcomes and optimized results. Multi-agent frameworks integrate various generative AI models and tools, creating intelligent systems capable of managing a range of tasks, from simple to highly complex. This versatility is crucial in dynamic business environments where adaptability is key.
A key advantage of multi-agent systems is their increased flexibility and scalability, essential for adapting to changing business needs. Unlike single-agent systems, multi-agents can aggregate diverse AI/ML models and integrate with third-party tools, offering specialized solutions for specific challenges. These systems enable the creation of new types of intelligence capable of performing more complex tasks with better results. Furthermore, multi-agent systems enhance communication and collaboration among intelligent agents, leading to improved efficiency and outcomes for tasks requested by humans or other applications.
Multi-agent systems excel in a range of practical scenarios:
- Math Solving: Addressing complex mathematical problems where single large language models might struggle.
- Multi-Agent Coding: Assisting in software development processes, including user stories, coding, testing, and design.
- Conversational Interactions: Improving user-machine interactions as mediators in conversational AI.
- Business Process Automation: Automating corporate operational systems, enhancing customer experience, and surpassing legacy bots' limitations.
- Online Decision-Making: Enriching decision-making by utilizing various sources, such as documents and webpages.
- Retrieval-Augmented Generation: Enhancing text quality from LLMs by incorporating corporate knowledge, ensuring relevance and security.
Current Trends and Innovations in AI that Complement Multi-Agent Systems
AutoML represents a significant advancement in the field of AI, particularly impacting multi-agent systems. It automates the process of applying machine learning models to real-world problems, including selection, composition, and parameterization. By simplifying the machine learning process, AutoML has made these technologies more user-friendly, accessible to organizations without specialized ML expertise, and efficient in producing accurate outputs faster than traditional methods. It encompasses a wide range of tasks such as raw data processing, feature engineering, model selection, deployment, and result analysis. The integration of AutoML with multi-agent systems could significantly enhance their performance, making the development and implementation of complex AI solutions more efficient and cost-effective. AutoML's capacity for neural architecture search and transfer learning is particularly beneficial, as it helps discover new architectures and apply existing ones to novel problems, which is a crucial aspect for the dynamic nature of multi-agent systems.
Multi-modal agents, which are AI systems that can process and analyze data in various forms (text, images, audio, etc.), are becoming increasingly important in fields like financial markets. These agents use generative AI to analyze diverse data sources, providing comprehensive insights and enhancing decision-making processes. Multi-modal agents enable applications such as smart reporting, market intelligence, quantitative modeling, compliance, and fraud detection. They automate routine tasks and amplify the capabilities of professionals, such as financial analysts, to work more efficiently and accurately. In practice, these agents consolidate insights from a wide range of data types on a large scale, offering scalable and secure solutions within platforms like AWS. The technical architecture of these systems typically involves using various AI tools for processing different data types. This architecture illustrates the symbiotic relationship between multi-modal learning and multi-agent systems, where the latter can greatly benefit from the versatility and depth of insights provided by the former.
These trends and innovations highlight the evolving landscape of AI and its applications. The integration of AutoML and multi-modal learning into multi-agent systems represents a leap forward in efficiency, scalability, and effectiveness, opening new possibilities for complex problem-solving across various industries.
Practical Applications and Case Studies of Multi-Agent Systems
Multi-agent systems (MAS) are revolutionizing various industries with their advanced capabilities. The integration of multiple agents enables these systems to undertake complex tasks and improve decision-making processes. Here are some real-world applications and case studies demonstrating their impact:
1. Math Solving and Coding
- MAS have been applied effectively in math solving and multi-agent coding, showcasing a level of specificity and role diversification that single agents cannot match.
2. Conversational Interactions and Business Process Automation
- In conversational AI and business process automation, MAS enhance user-machine interactions and automate processes, thereby enhancing customer experiences and addressing challenges of legacy systems.
3. Online Decision-Making
- MAS are utilized for online decision-making, integrating information from various sources to inform decisions.
4. Supply Chain Management
- In supply chain management, MAS optimize the flow of goods and materials, predict demand, assign orders, and improve the sales process.
5. Enhanced Customer Experience
- MAS contribute to personalized customer experiences, significantly boosting customer satisfaction and loyalty.
These examples underscore the versatility and efficiency of multi-agent systems in addressing complex challenges across various sectors, making them a vital component in the contemporary technological landscape.
Future Outlook and Potential Challenges of Multi-Agent Systems
The future of multi-agent systems (MAS) is poised for deeper integration into our interconnected, networked world, where they will increasingly interact with both humans and other agents. Key advancements will involve novel designs, models, and methods in computer-agent representations and decision-making processes, tailored to accommodate human interactions and societal contexts.
Challenges in MAS implementation include:
- Technical Challenges: Developing realistic human decision-making models necessitates significant changes in traditional AI models, requiring new methods and designs that integrate human reasoning aspects.
- Ethical and Societal Challenges: The expanding role of MAS prompts important ethical and societal considerations, particularly in contexts where they interact closely with humans.
- Collaborative Dynamics: Future developments are expected to focus on algorithms that optimize the combined performance of humans and agents, requiring a balance between human and computer agent preferences and capabilities.
AI advancements are likely to significantly influence MAS evolution. The focus will be on enhancing collaboration between humans and AI agents to achieve combined performance beyond individual capabilities. This involves the integration of insights from social sciences into the design of MAS for effective human-agent collaboration.
The future of MAS is promising, yet it demands a multidisciplinary approach integrating technical, ethical, and societal considerations for safe and effective implementation.
Conclusion
In summary, multi-agent systems (MAS) represent a significant advancement in the field of artificial intelligence, offering unparalleled flexibility, scalability, and efficiency. These systems, by coordinating multiple AI entities, excel in complex environments, addressing challenges that single-agent systems cannot. The integration of technologies like Automated Machine Learning (AutoML) and multi-modal learning further enhances their capabilities. Real-world applications across industries like finance, healthcare, and supply chain management demonstrate their transformative potential. Looking ahead, the evolution of MAS will be driven by continuous AI innovations, albeit with technical, ethical, and societal challenges. Their role in modern AI applications is crucial, driving innovation and efficiency across various sectors and reshaping the future of AI-driven solutions.