The Role of Multimodal Communication in Enhancing AI Interactions
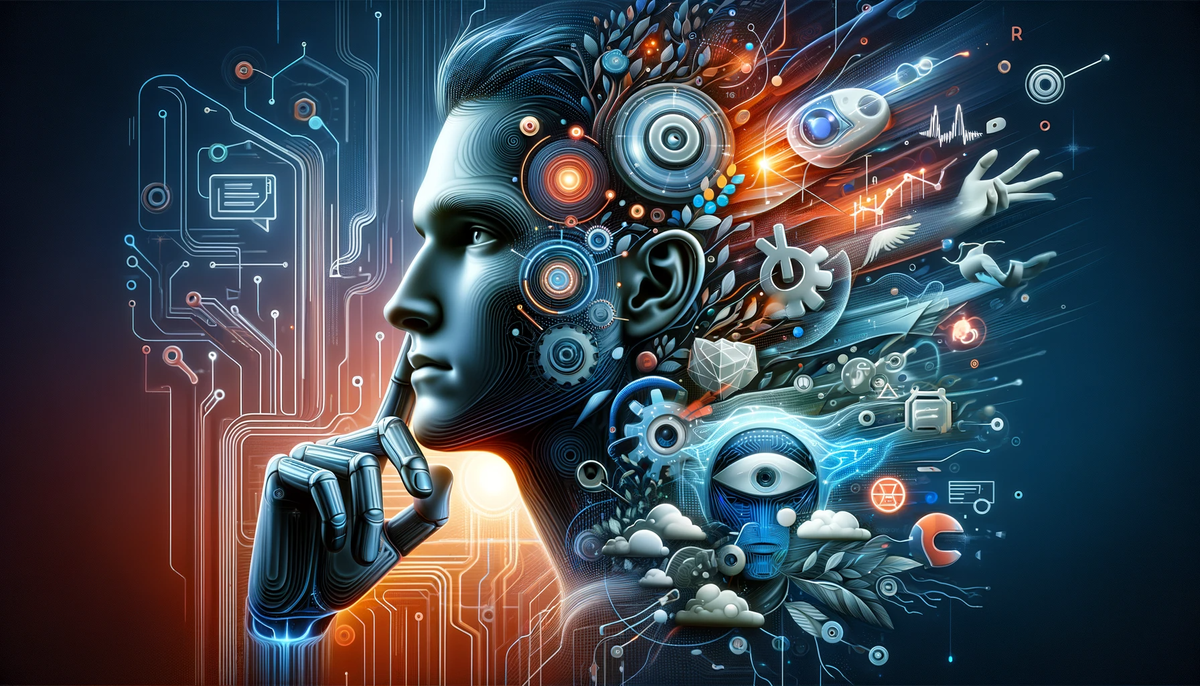
Flexible Interfaces
In the field of artificial intelligence (AI), multimodal communication is a major direction for development. This approach involves systems that can process and integrate inputs and outputs from multiple modalities, such as text, images, and sounds. By doing so, these systems create interactions that are more dynamic and intuitive, more closely mirroring the multimodal richness of human communication. Multimodal AI is a quite useful advance over systems that rely on a single modality such as text.
The architecture of multimodal AI typically has three key components:
- Input Module: This module includes separate unimodal neural networks that process different types of data.
- Fusion Module: This module integrates information from the various input networks using methods ranging from simple concatenation to more complex attention mechanisms.
- Output Module: The final stage generates outputs based on the fused data.
Recent Advances
2023 has witnessed notable advancements in multimodal AI.
OpenAI's DALL-E 3, integrated within ChatGPT, combines the capabilities of a large language model (LLM) with an image generation system. DALL-E 3 creates detailed, high-resolution images from textual descriptions, taking into account both context and artistic nuance. This integration allows users to provide textual prompts, to which DALL-E 3 responds with visually coherent interpretations. The model can generate various artistic styles and handle a wide range of requests.
Microsoft's KOSMOS-2 focuses on processing both text and images. It integrates "multimodal grounding," generating precise image captions and reducing errors common in previous LLMs. Additionally, its "referring expression generation" feature enhances user interactions with visual content by allowing specific queries about image regions.
Google's Gemini is trained on a variety of data types including images, code, audio, and video, allowing it to directly perceive and interact with the physical world in a more comprehensive manner. This makes it useful in fields such as manufacturing, e-commerce, and agriculture.
Google's Mirasol3B is designed to handle long video sequences effectively. It uses an autoregressive model to process time-aligned modalities like audio and video, and a separate component for unaligned context modalities such as text. Its architecture allows for processing of extended audio and video inputs, making it suitable for applications that involve lengthy video content.
Meta AI's Omnivore is distinctive for its ability to handle 3D data along with other visual modalities. It processes images, videos, and 3D data using the same parameters.
Meta AI's FLAVA is a foundational model trained for over 35 multimodal tasks, including image recognition, text recognition, and joint text-image tasks. This model can process a diverse range of tasks using a single, jointly trained model.
Meta AI's CM3 is an open-source model capable of generating new images and captions, as well as filling in larger structured text sections. It is trained on structured multimodal documents and can adapt to various tasks using the same model architecture.
Meta AI's data2vec is a self-supervised learning model that achieves state-of-the-art results in speech, vision, and text recognition. It uses a unified approach to training across different modalities with versatile handling of diverse data types.
Practical Applications
The applications of multimodal AI span diverse fields, each harnessing its capability to interpret multiple data types.
- In healthcare, multimodal AI can improve medical imaging analysis, disease diagnosis, and treatment planning, leading to better patient outcomes.
- Retailers can use multimodal AI for personalized recommendations and optimizing product searches, enhancing customer satisfaction and loyalty.
- In agriculture, integrating satellite imagery, weather data, and soil sensor data with multimodal AI can optimize farming practices and increase crop yields.
- Manufacturing can benefit from multimodal AI in quality control, predictive maintenance, and supply chain optimization, improving efficiency and reducing waste.
- The entertainment industry can use multimodal AI to analyze emotions and speech patterns, aiding content creators in tailoring their offerings to specific audiences.
- In social robotics, multimodal human-robot interaction (HRI) employs various modalities like voice, image, text, eye movement, and touch.
What's Coming Up?
AI is heading towards more interactive, multimodal systems. These systems will interact across multiple modalities to yield a more dynamic and intuitive user experience. Such advances will transition the field from generative to interactive AI, where AI will interact with users more naturally, mimicking human-like conversations.
Interactive multimodal AI will be useful across many professional fields. In business, these systems could transform spreadsheets and charts into dynamic, interactive content, assisting decision-making processes. AI will thereby become better positioned to aid complex analytical tasks. Overall, multimodal AI will bring AI closer to the fluidity and richness of human communication.