Autonomous Decision-Making with Agentic AI
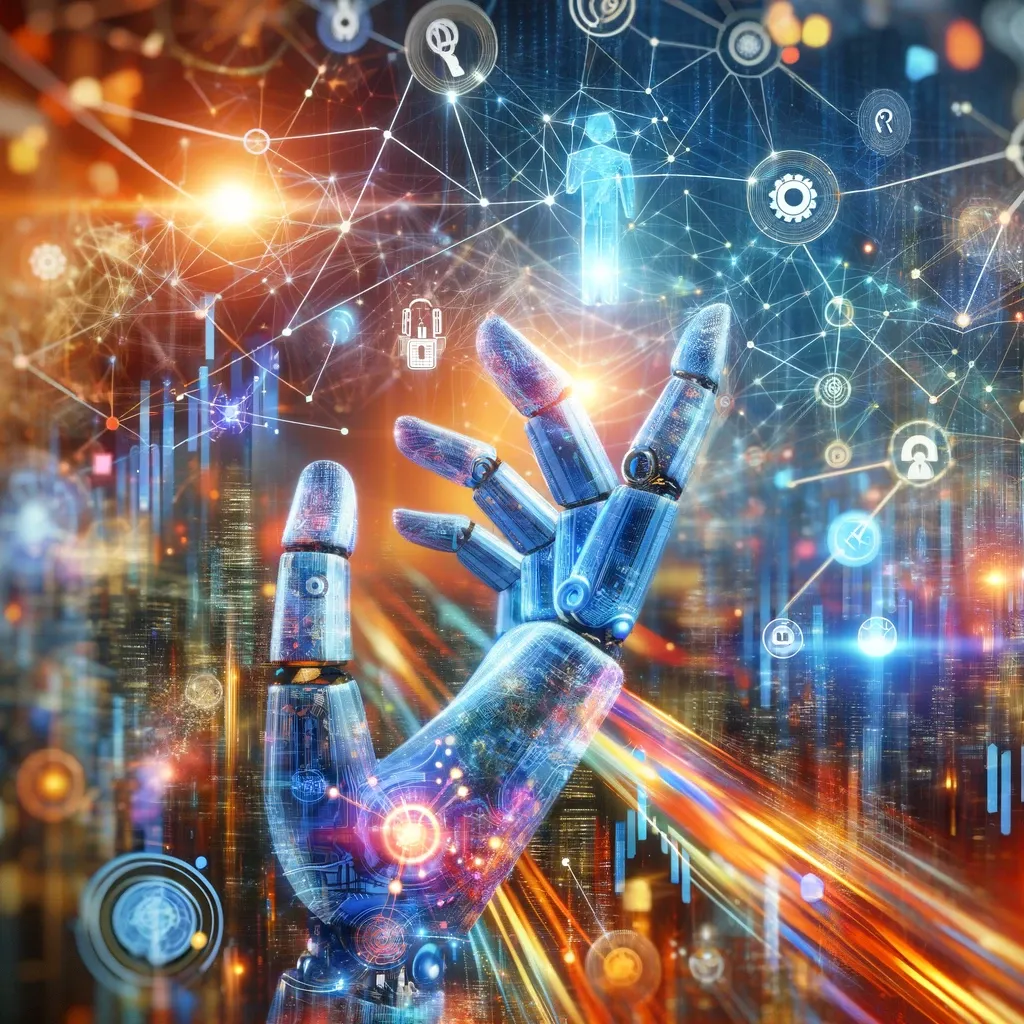
An intelligent supply chain management system detects an imminent weather event threatening to disrupt a delivery route. Instead of merely alerting human operators, it autonomously evaluates alternative routes and suppliers, calculates the cost and time implications, and reroutes shipments to avoid delays. This system isn't just following a script; it's making a complex decision based on real-time data and executing it to mitigate risks.
Consider a system that autonomously resolves a reported product issue in customer service. It analyzes the product's warranty status, the nature of the problem, and the customer's history. Based on this analysis, it decides whether to offer a replacement, repair, or refund and then takes the necessary actions. This process involves nuanced decision-making that aligns with business rules and customer satisfaction goals.
These examples illustrate the capabilities of agentic artificial intelligence (AI), which go beyond routine task automation and Q&A chatbots (e.g., ChatGPT). Agentic AI systems are characterized by their ability to make independent decisions and take actions based on real-time analysis and strategic assessment, at par with or surpassing the decision-making process of experienced managers.
Agentic AI is not a futuristic idea but a reality in forward-thinking organizations. It will soon become the standard for successful businesses. Understanding agentic AI is crucial for unlocking its potential in automation and decision-making. Mastering its principles is essential for ethical, effective AI solutions. Security, privacy, and regulatory concerns must also be addressed.
What is Agentic AI?
Agentic AI refers to AI systems with the capacity for autonomous decision-making and action. These systems go beyond executing programmed instructions; they can assess situations, make judgments, and initiate actions independently or in collaboration with human participants. Agentic AI is characterized by its ability to perceive the environment, process information, derive insights, and determine the best course of action without human intervention. This involves a combination of advanced machine learning, data analytics, and cognitive computing capabilities, enabling the AI to act with a degree of agency similar to that of a human decision-maker.
Agentic AI systems are differentiated from other AI systems by their autonomy, decision-making capabilities, and the ability to act upon those decisions without human intervention. Here are the key characteristics that set agentic AI apart:
Autonomy: Unlike conventional AI, which operates under strict guidelines and requires human input for decision-making, agentic AI can function independently, assessing situations, and making choices without external direction.
Proactive Decision-Making: Agentic AI systems proactively make decisions based on their analysis and understanding of the data rather than merely reacting to specific commands or executing predefined tasks.
Adaptability: These systems can adapt to changes in their environment or operational context, learning from new information and experiences to refine their decision-making processes.
Contextual Understanding: Agentic AI has a sophisticated ability to understand and interpret context, allowing it to make decisions that are nuanced and situationally appropriate.
Goal-Oriented Actions: They are designed to pursue specific goals or objectives, taking necessary actions to achieve those outcomes effectively.
Continuous Learning: Unlike static AI models, agentic AI systems continuously learn from their interactions and experiences, enhancing their capabilities and performance over time.
These characteristics enable agentic AI to operate more like a human in its ability to assess, decide, and act, marking a significant evolution from traditional AI systems.
Evolution of Agentic AI
The concept of agentic AI has its roots in the early days of artificial intelligence research, which began in the 1950s. Pioneering figures like Alan Turing and John McCarthy envisioned intelligent machines capable of independent thought and action. However, initial AI systems were designed for specific tasks like playing chess or solving math problems, operating on predefined algorithms and lacking the ability to learn or make independent decisions. As technology progressed, the limitations of these rule-based systems became apparent, leading to the development of machine learning and neural networks in the late 20th century. These advancements allowed AI to learn from data and improve over time, paving the way for a more autonomous future.
The transition from task-specific AI to decision-making entities began in earnest with the advent of machine learning and the expansion of computational power in the late 20th and early 21st centuries. Researchers like Yann LeCun, Geoffrey Hinton, and Yoshua Bengio made significant contributions in developing algorithms that could not only learn from data but also make predictions and decisions based on their learning. This shift marked the beginning of the agentic AI era, where systems were designed to act independently, rather than just perform pre-programmed tasks.
The integration of natural language processing (NLP) and generative AI significantly enhanced the capabilities of these systems. Pioneering research in NLP by Joseph Weizenbaum and his successors facilitated a better understanding and interpretation of human language. This enabled AI to interact more naturally and make informed decisions based on textual data. Meanwhile, the work of researchers like Ian Goodfellow on generative adversarial networks (GANs) allowed AI systems to create new content and predict outcomes, enriching the decision-making process with innovative solutions and strategies.
The 2010s saw a significant acceleration in the development of agentic AI, driven by advancements in deep learning, big data analytics, and the emergence of large language models (LLMs) like GPT-3. These technologies, spearheaded by researchers like Geoffrey Hinton and Andrej Karpathy, enabled AI systems to understand complex patterns, make decisions, and act in ways that mimicked human cognitive processes. For example, autonomous vehicles powered by agentic AI are now capable of navigating complex traffic situations with minimal human intervention, while AI in fields like finance and manufacturing are making investment decisions and optimizing production lines based on real-time data analysis.
The current stage of agentic AI is characterized by its integration into various sectors, where it not only makes decisions but also learns from its environment and continuously improves its decision-making capabilities. These systems are now capable of handling complex, multi-faceted problems in real time, adapting to new information, and making decisions that consider a wide range of factors, including ethical and regulatory considerations.
The progression of agentic AI mirrors a broader paradigm shift in AI, transitioning from systems designed to execute specific, pre-programmed tasks to entities endowed with a degree of autonomy and decision-making abilities akin to those possessed by humans. This advancement has been facilitated by the convergence of various technological advancements, including machine learning, data analytics, augmented computational capabilities, and the intricate language comprehension afforded by NLP and LLMs. These elements have synergistically paved the way for AI systems to operate independently and effectively in diverse contexts, signifying a substantial evolution from conventional AI to the more dynamic and autonomous agentic AI.
Applications of Agentic AI
Agentic AI is rapidly transforming industries by offering autonomous solutions that enhance efficiency, decision-making, and operational workflows. Let's delve into some of its most impactful applications:
- Self-Healing IT Infrastructure: Imagine IT systems that not only monitor themselves but also autonomously troubleshoot problems and respond to security threats. Agentic AI systems make this a reality. They can analyze network traffic, identify anomalies, and initiate countermeasures against cyberattacks, all without human intervention. This significantly reduces downtime and strengthens overall security posture. Companies like Palo Alto Networks are at the forefront of developing such AI-powered cybersecurity solutions.
- Personalized Healthcare Delivery: The healthcare sector is witnessing a significant impact from agentic AI. AI systems can analyze vast amounts of medical data, including patient records, scans, and treatment histories, to assist doctors in diagnosing diseases, predicting potential complications, and even recommending personalized treatment plans. This can lead to more accurate diagnoses, improved patient outcomes, and reduced healthcare costs. IBM's Watson Oncology platform exemplifies this potential by using AI to analyze medical data and suggest treatment options tailored to each patient's specific needs.
- Predictive Maintenance in Manufacturing: Agentic AI is transforming the manufacturing landscape by enabling predictive maintenance and real-time process optimization. AI systems can monitor equipment performance, predict potential failures before they occur, and autonomously adjust production parameters to ensure efficiency and minimize downtime. General Electric's Predix platform is a prime example, leveraging industrial IoT sensors and AI to predict equipment failures and optimize maintenance schedules in manufacturing facilities.
- Intelligent Supply Chain Management: Gone are the days of static, reactive supply chains. Agentic AI revolutionizes supply chain management by enabling autonomous decision-making and real-time data analysis. AI systems can analyze everything from inventory levels to transportation routes and weather patterns. Based on this analysis, they can autonomously optimize logistics, reroute shipments to avoid disruptions, and ensure timely delivery of goods. Companies like UPS and FedEx are actively integrating agentic AI solutions to optimize their logistics networks and enhance supply chain efficiency.
- Personalized Customer Service at Scale: Agentic AI chatbots are transforming customer service by providing 24/7 availability, personalized interactions, and efficient issue resolution. These AI-powered chatbots can understand customer queries, answer questions, troubleshoot problems, and even anticipate future needs. This not only improves customer satisfaction but also reduces the workload on human customer service representatives. Sephora's use of AI chatbots to answer customer product inquiries and offer personalized recommendations is a notable example.
The road ahead
As we explore the depths of agentic AI's capabilities, it becomes apparent that this technological advancement extends beyond mere automation. It represents a transformative force poised to reshape entire industries. Imagine AI-powered systems collaborating with scientists to unlock unprecedented medical breakthroughs or working in tandem with engineers to design sustainable and resilient infrastructure solutions. In finance, agentic AI empowers financial institutions with advanced fraud detection capabilities and personalized banking experiences.
Agentic AI represents a groundbreaking advancement in computer science, elevating machines to unprecedented levels of capability. This type of AI possesses remarkable intelligence, enabling it to engage in learning, adaptation, and independent decision-making. Think of it as a highly competent assistant capable of helping solve complex global challenges.
However, it is imperative to approach the development and deployment of agentic AI with utmost prudence. Careful consideration must be given to the intended applications of this technology, ensuring that they align with the well-being of society as a whole. It is essential to avoid the monopolization of agentic AI by a select few individuals or organizations and instead strive for equitable access and benefits for all.
Agentic AI and Dragonscale
In the upcoming weeks, we will share our experiences, ideas, and code related to agentic AI, focusing on our open-source framework, Rustic AI. Rustic AI enables the creation of agentic AI solutions by leveraging multi-modal architecture. It combines well-established concepts and experiences in building distributed, decoupled systems with the latest advancements in AI and NLP. If you are eager to learn more about agentic AI and how you can develop your own agentic AI solutions, be sure to subscribe to our newsletter for updates.